Introduction
In the landscape of artificial intelligence, the emergence of explainable AI (XAI) marks a pivotal shift towards transparency and accountability. This blog explores the critical nature of XAI, emphasizing its role in demystifying AI’s decision-making processes. As AI systems increasingly influence various sectors, the ability to interpret and understand these models becomes crucial, especially in areas where decisions have significant impacts.
Delving into the Essence of Explainable AI
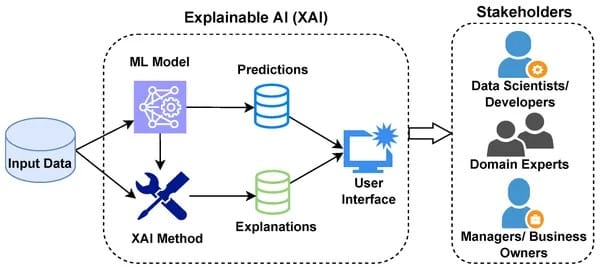
- Explore the importance of XAI in fostering trust and transparency in AI applications.
- Discuss the impact of XAI on sectors where AI’s decisions are critical, highlighting the shift towards more interpretable AI systems.
Explainable AI (XAI) represents a transformative shift in the field of artificial intelligence, aiming to bridge the gap between advanced AI algorithms and human understanding. At its core, explainable AI seeks to unravel the complex decision-making processes of AI models, making them transparent and comprehensible to users. This endeavor is not merely about technical transparency but also about building trust and reliability in AI systems across various domains. By providing clear insights into how AI models arrive at their conclusions, XAI enables stakeholders to validate and trust AI-driven decisions, fostering a collaborative synergy between humans and machines.
The essence of explainable AI goes beyond algorithmic transparency; it’s about creating a dialogue between AI and its users. By demystifying AI operations, XAI empowers users to gain insights into the rationale behind AI decisions, ensuring that these systems are used responsibly and ethically. This is particularly crucial in sectors where AI’s impact is profound, necessitating a level of interpretability that aligns with human values and ethical standards. As AI continues to permeate diverse sectors, the role of XAI becomes increasingly vital, ensuring that AI advancements are aligned with societal norms and expectations.
Understanding Explainable AI: A Global Benchmark
The principles of explainable AI are setting new standards in the AI community, advocating for models that are not only effective but also interpretable. This section outlines the core concepts of explainable AI, its significance in the AI ecosystem, and the global movement toward integrating transparency in machine learning.
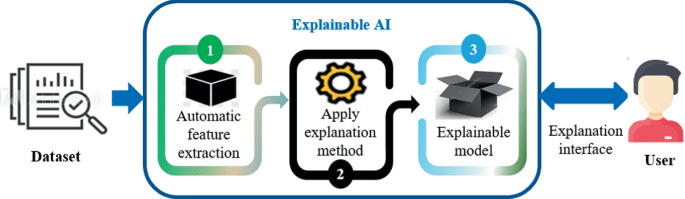
The Core Concepts of Explainable AI
The core concepts of Explainable AI revolve around interpretability, transparency, and accountability. Interpretability refers to the extent to which a human can understand the cause of a decision made by an AI system. This understanding is crucial for validating the model’s performance and ensuring its alignment with ethical and legal standards. Transparency in AI, meanwhile, involves clear communication of the model’s functionality, limitations, and decision-making processes, enabling users to comprehend and trust AI systems.
- Define what XAI entails and its critical components.
- Illustrate the global trend towards adopting XAI in AI development and deployment.
Accountability is another cornerstone of explainable AI, ensuring that AI systems are designed and deployed in a manner that allows for responsibility and recourse in case of adverse outcomes. This involves creating AI models that not only make accurate predictions but also provide explanations that are meaningful and actionable for end-users. By embedding these core concepts into AI development, XAI aims to create a foundation for AI systems that are not only powerful and efficient but also aligned with human-centric values, facilitating a responsible integration of AI into society.
Techniques and Approaches in XAI
Explainable AI encompasses various techniques and methodologies to make AI decisions transparent. This part delves into the different approaches to achieving explainability in AI, from model-agnostic methods to intrinsic interpretability, providing a comprehensive overview of how AI models can be made understandable.
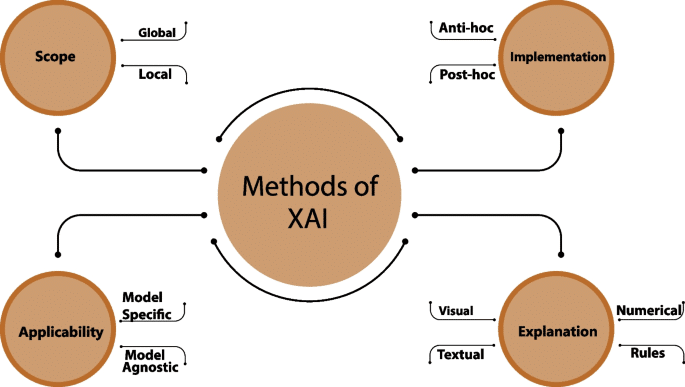
Interpretable Models and Techniques
- Discuss various techniques that make AI models interpretable, such as feature importance scores and decision trees.
- Explore different approaches to explainability, including post-hoc explanations and transparent model design.
Interpretable models and techniques in explainable AI are designed to provide insights into the decision-making processes of AI, offering clarity on how input data is transformed into outputs. Techniques like feature importance analysis help in identifying which aspects of the data are influencing the model’s decisions, providing users with a clear understanding of the model’s behavior. Similarly, decision tree models offer a straightforward, rule-based approach to decision-making, where the path from input to decision can be easily traced and understood.
Another technique, model-agnostic methods, allows for interpretability regardless of the underlying model complexity. These methods, including partial dependence plots and individual conditional expectation plots, provide visualizations and statistical measures that elucidate the relationship between input features and the model’s predictions. By employing these interpretable models and techniques, data scientists can create AI systems that not only excel in performance but also in providing transparent and understandable explanations, enhancing the user’s trust and confidence in AI applications.
XAI in Practice: Industry Applications
XAI is not just a theoretical concept but is being actively implemented across various industries. This section highlights practical applications of XAI in fields like healthcare and finance, showcasing how interpretability in AI is enhancing decision-making processes and outcomes.
Sector-Specific Applications of explainable AI
In healthcare, XAI is revolutionizing how medical professionals interpret AI-driven diagnostics and treatment recommendations. By providing interpretable models, clinicians can understand the rationale behind AI-generated insights, aligning them with clinical expertise and patient care standards. For instance, in diagnostic imaging, XAI can highlight the features within an image that led to a particular diagnosis, aiding doctors in making informed decisions.
In the financial sector, XAI is being employed to bring transparency to automated trading, credit scoring, and risk assessment. Financial institutions leverage XAI to explain credit decisions to customers, ensuring compliance with regulatory requirements and building trust. By elucidating the factors influencing AI-driven financial advice or risk evaluations, XAI helps in demystifying complex financial models, enabling stakeholders to make more informed and confident decisions based on AI insights.
- Provide examples of XAI in healthcare, such as interpretable models in diagnostics.
- Discuss the application of XAI in finance, focusing on transparent credit scoring systems.
Challenges and Future Directions in XAI
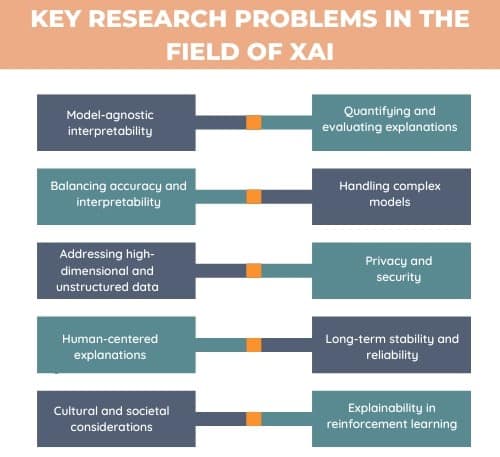
While XAI offers numerous benefits, it also faces challenges and limitations. This part addresses the hurdles in implementing XAI and speculates on future advancements, offering insights into how XAI is expected to evolve and shape the future of AI.
Overcoming XAI Challenges
One of the primary challenges in XAI is balancing the trade-off between model complexity and interpretability. Highly complex models, such as deep neural networks, offer superior performance but often at the cost of interpretability. Developing methodologies that retain the model’s performance while enhancing its transparency is a key focus area in XAI research. Addressing this challenge requires innovative approaches that do not compromise on the model’s accuracy or the quality of explanations provided.
- Address the technical and ethical challenges in implementing XAI.
- Speculate on future advancements in XAI and their potential impact on AI development.
Another significant challenge is ensuring that the explanations generated by XAI are meaningful and useful to end-users, who may not have technical expertise. This involves designing explanation interfaces and visualization tools that convey the model’s decision-making process in an intuitive and accessible manner. Overcoming these challenges is crucial for the widespread adoption of XAI, as it ensures that the benefits of AI are accessible to all, fostering an environment where AI’s decision-making processes are not just transparent but also aligned with the broader goals of society and individual user needs.
Conclusion
Conclude by reinforcing the importance of explainable AI in the modern digital world, emphasizing how XAI is crucial for ethical AI practices, enhancing user trust, and ensuring transparency in AI’s decision-making processes. Encourage the AI community to continue advancing XAI to foster a more accountable and transparent future for AI technologies.
The imperative for explainable AI extends beyond the realm of enhancing user trust and facilitating understanding. It is a foundational element in the ethical deployment of AI technologies, ensuring that decisions made by AI are not only justifiable but also aligned with societal values and norms. As AI systems become more autonomous and their decisions more impactful, the need for accountability and transparency becomes paramount.
XAI serves as a bridge between the complex inner workings of AI models and the practical, ethical considerations of their outputs, ensuring that AI technologies remain a force for good, augmenting human capabilities without obscuring the logic behind their actions.
Moreover, the advancement of XAI is a collaborative endeavor that requires the concerted effort of the entire AI community. Researchers, developers, ethicists, and users must work together to push the boundaries of what is possible in AI, making it more interpretable, accountable, and aligned with human values. This commitment to advancing XAI will ensure that AI’s integration into society enhances, rather than obscures, our understanding of the world, fostering an era of technology that is both advanced and inherently aligned with the principles of ethical AI.
You have brought up a very superb points, thankyou for the post.